Introduction to ProteinPaint
ProteinPaint is a tool made available as part of the PeCan Data Portal. The principle goal of this data portal is to facilitate exploration of childhood cancer genomics data. However, some tools, such as ProteinPaint are generally useful for visualizing the recurrence of any set of variants in a gene in the context of protein domains and other information.
This section will provide a brief introduction to ProteinPaint’s features and demonstrate its use with a few examples and exercises.
The tool is entirely web based. First navigate to the tool’s homepage: ProteinPaint. Note that it has its own tutorials.
Guided tour of pre-loaded data
Go through the following exercise to explore the functionality of this resource:
- Choose an example gene to explore (e.g. EGFR). Enter it in the search box and select it from the drop down of suggestions.
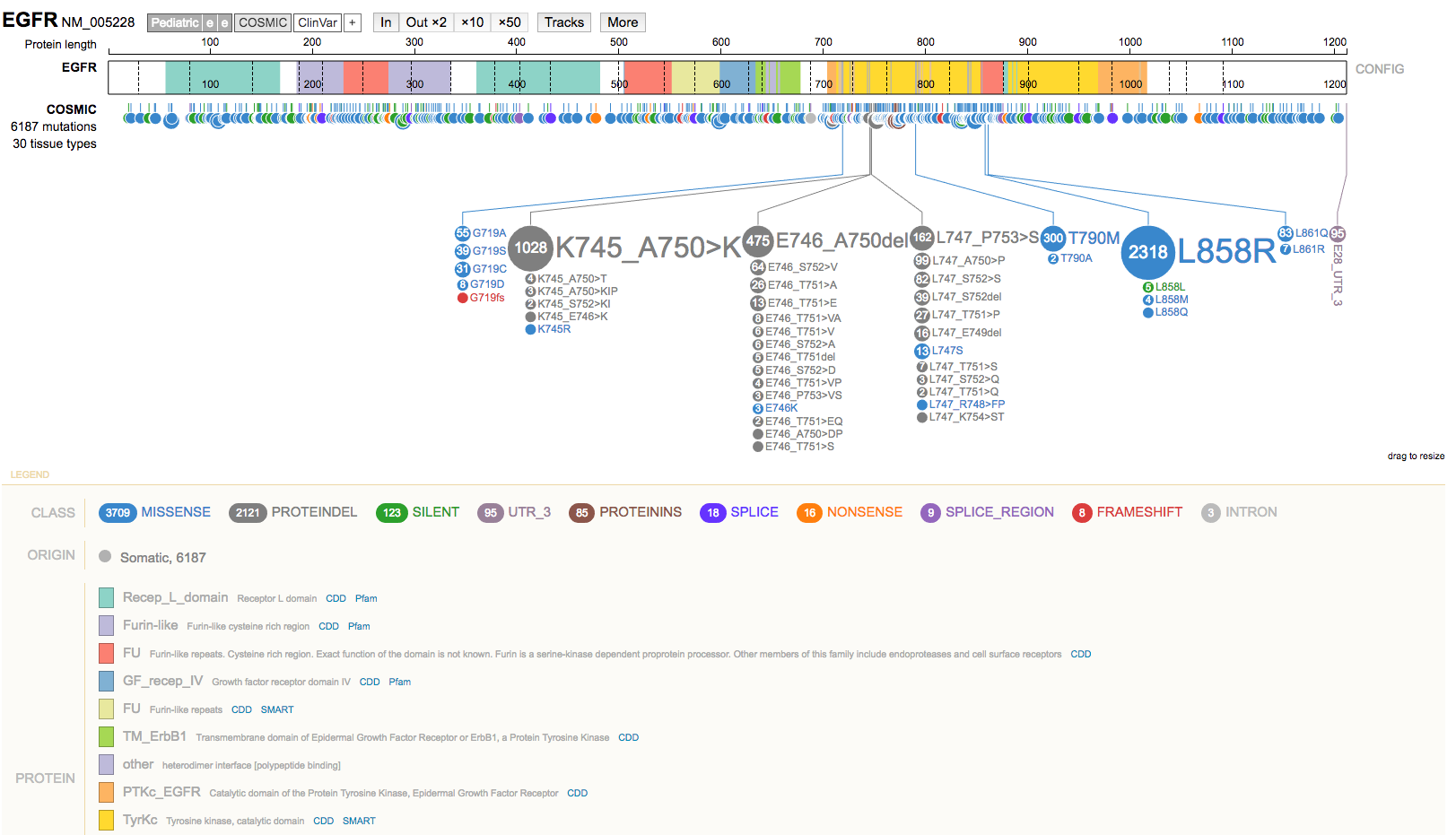
- Select the gene name (top left) to view a summary of the gene.
- Use the
CONFIG
option (right side) to change between display modes by selectingswitch display mode
. Try theSplicing RNA
option. Note these options are also available from the gene summary view mentioned above.
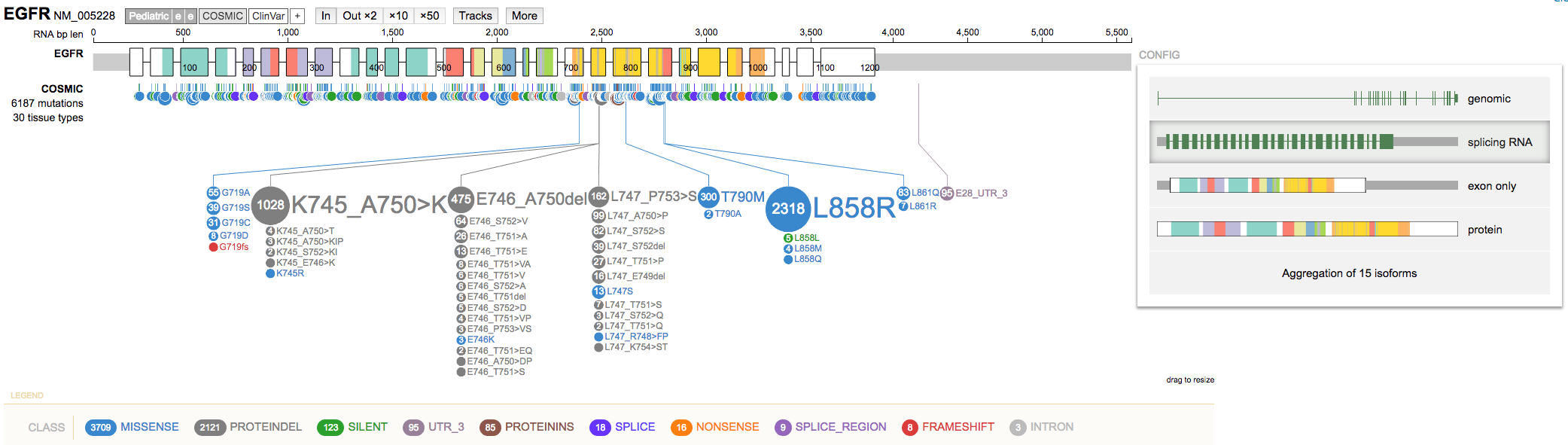
- Use the
Tracks
button to view the list of available tracks and turn on theRefGene
track.
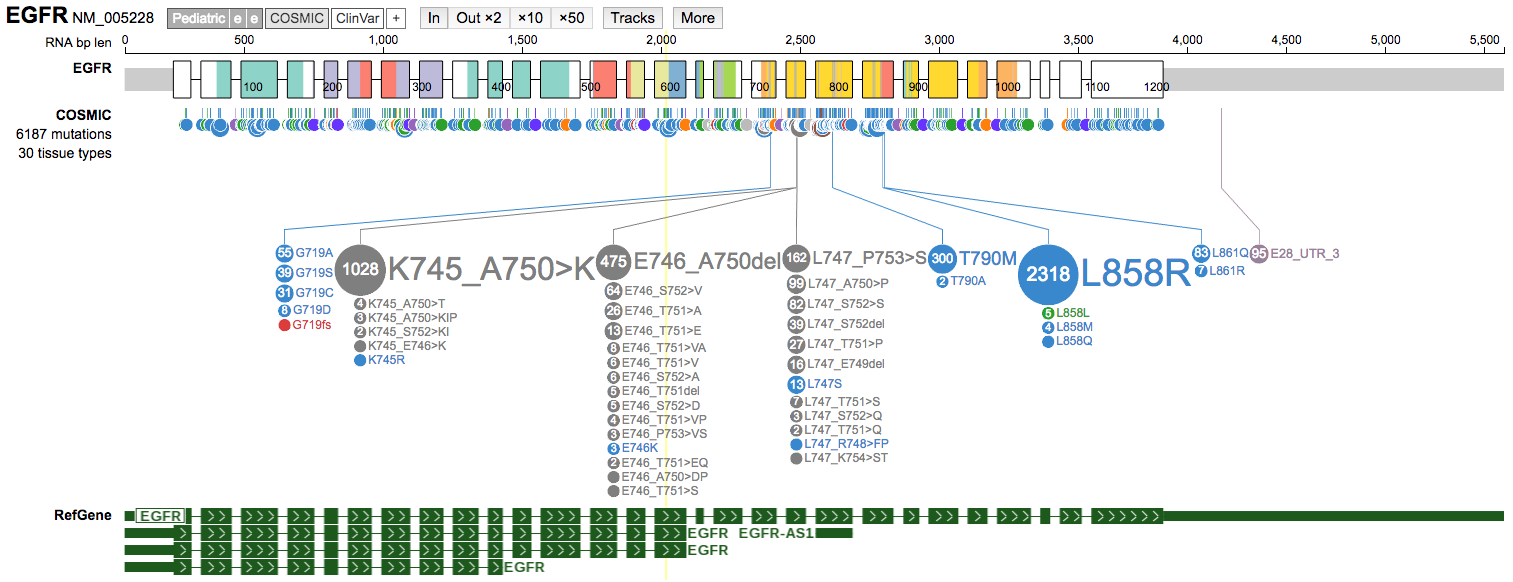
-
Use the
CONFIG
menu to changeswitch display mode
back toProtein
. Also turn off theRefGene
track. - Select pre-loaded data to display (Pediatric, COSMIC, and ClinVar). Select COSMIC for this example. Use the
ABOUT
popup to learn more about each data source. - Zoom
In
on a mutation hotspot and navigate around that region. Once done, Zoom Outx50
to return to a view of the entire gene.
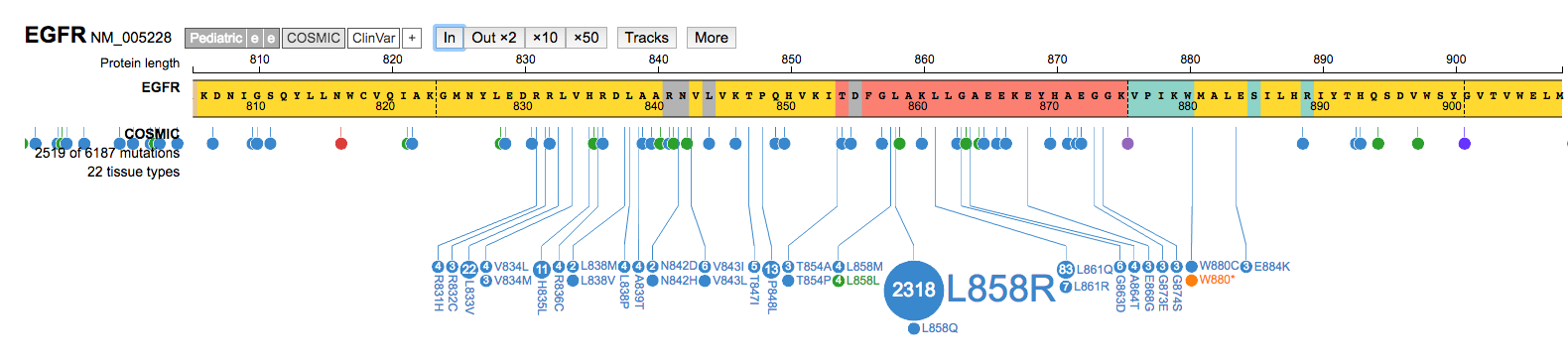
- Under the
More
menu, trySelect a region to highlight
. Select a region containing a hotspot mutation. Change the highlight color using the same menu.
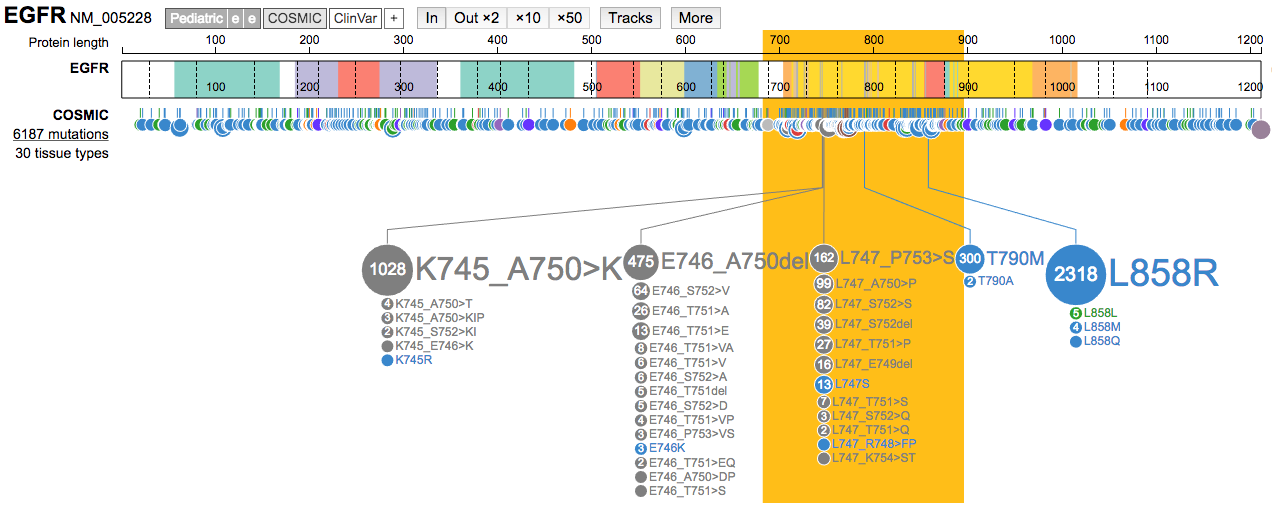
- Clear the highlighted region. Now use the
+ add protein domain
button (bottom left) to add a custom domain of interest. e.g.MUTATION HOTSPOT ; 700 900; red
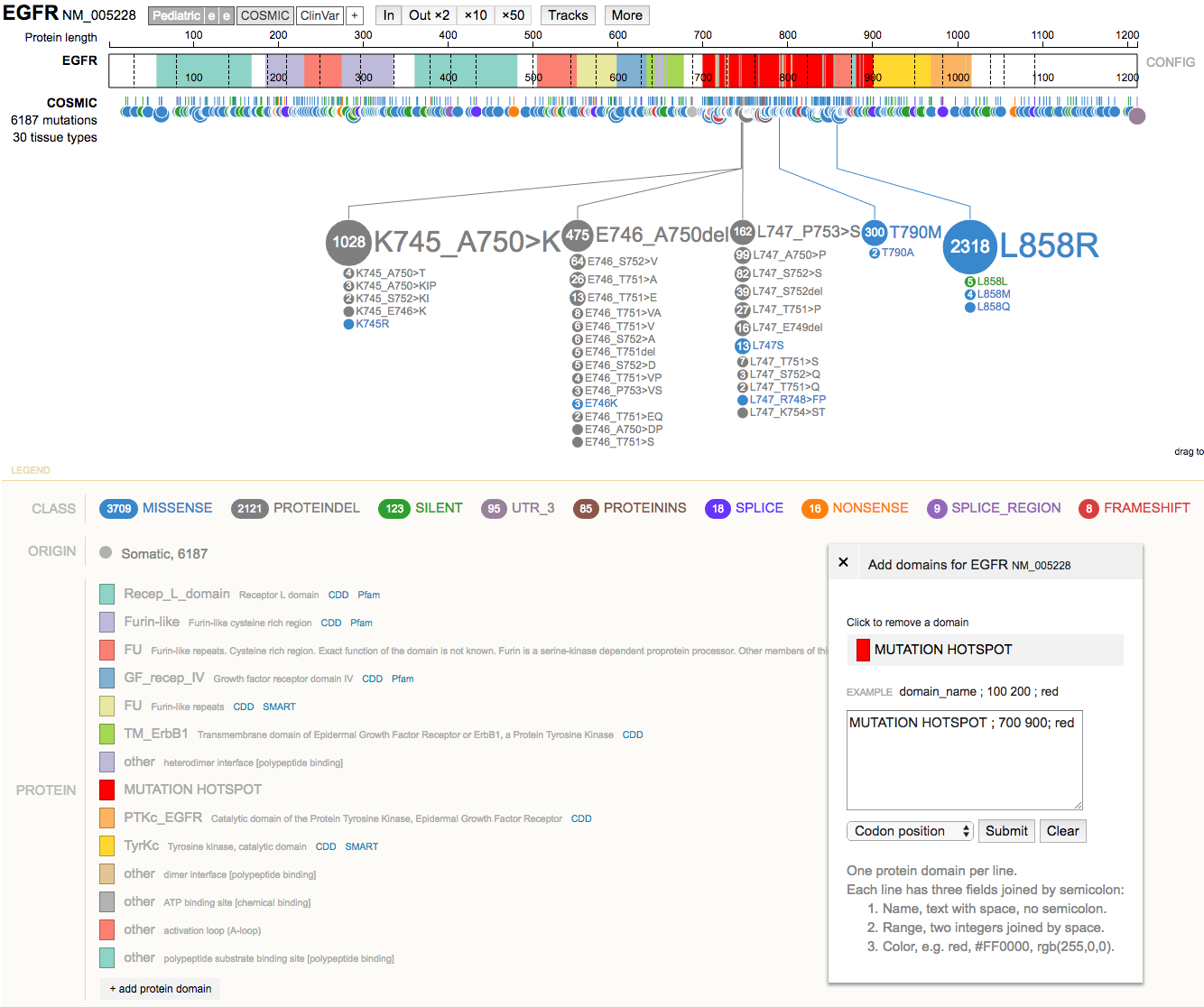
- Mouse over the gene diagram to view details on individual domains and amino acid positions.
- Select specific variants to learn more about what diseases they occur in. For example, load ERBB2 and the COSMIC data track. Two of the most common mutations are S310F and L755S. Select both of these. Which is more common in breast cancer? Use the
List
option to learn more about individual variant observations.
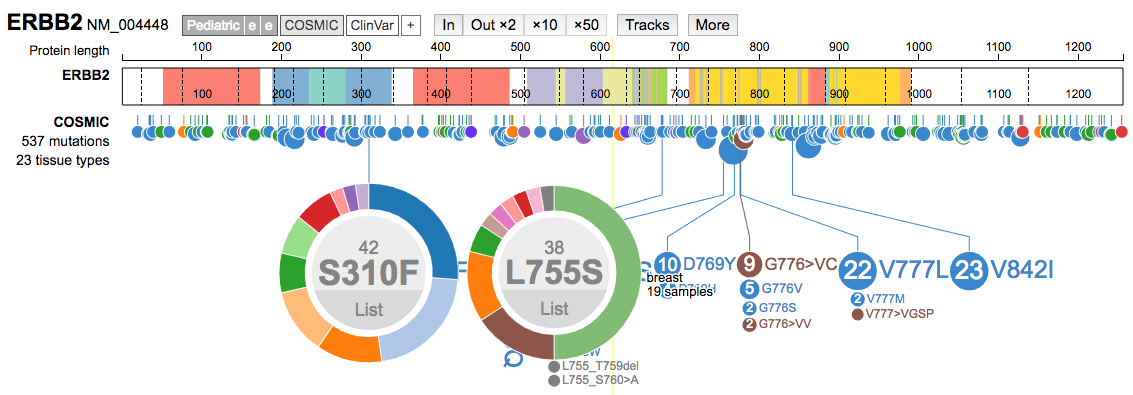
Importing custom data
-
Suppose we want to load a custom data set of our own variants into ProteinPaint. For illustration, we will use CIViCs list of VHL variants. To get that list go to www.civicdb.org, select
SEARCH
, select theVariants
tab, create a query whereGene
is
VHL
, and hit theSearch
button. Once the query completes, use theGet Data
option toDownload CSV
. Save this file. Rename it to CIViC-VHL-Variants.csv. We’ll need to reformat the data before inporting it into ProteinPaint. -
Open this file in R and perform the clean-up as follows:
# Load the data downloaded from CIViC
setwd("~/Downloads/")
dir()
x = read.csv(file = "CIViC-VHL-Variants.csv", as.is=1:4)
# Store only the variant names that we will parse for protein coordinates
vhl_variants1 = x[,2]
# Tidy up the names to remove the c. notations
vhl_variants2 = gsub("\\s+\\(.*\\)", "", vhl_variants1, perl=TRUE)
# Remove complex expressions beyond the "fs" in some variants
vhl_variants3 = gsub("fs.*", "fs", vhl_variants2, perl=TRUE)
# Limit to only those variants with a format like: L184P
vhl_variants4 = vhl_variants3[grep("^\\w+\\d+\\w+$", vhl_variants3, ignore.case = TRUE, perl=TRUE)]
# Remove variants with an underscore
vhl_variants5 = vhl_variants4[grep("_", vhl_variants4, ignore.case = TRUE, perl=TRUE, invert = TRUE)]
# Store the variant names for later
vhl_variant_names = vhl_variants5
# Extract the amino acid position numbers
vhl_variant_positions = gsub("\\D+(\\d+)\\D+", "\\1", vhl_variants5, perl=TRUE)
# Create a variant types list
# M=MISSENSE, N=NONSENSE, F=FRAMESHIFT, I=PROTEININS, D=PROTEINDEL, S=SILENT
types = vector(mode = "character", length = length(vhl_variant_names))
types[1:length(vhl_variant_names)] = "M"
types[grep("\\*", vhl_variant_names, ignore.case = TRUE, perl=TRUE)] = "N"
types[grep("fs", vhl_variant_names, ignore.case = TRUE, perl=TRUE)] = "F"
types[grep("ins", vhl_variant_names, ignore.case = TRUE, perl=TRUE)] = "I"
types[grep("del", vhl_variant_names, ignore.case = TRUE, perl=TRUE)] = "D"
# Store the values we care about in a new data frame
vhl_variants_final = data.frame(vhl_variant_names, vhl_variant_positions, types)
head(vhl_variants_final)
# Create the final format strings requested for ProteinPaint of the form: R200W;200;M
format_string = function(x){
t = paste (x["vhl_variant_names"], x["vhl_variant_positions"], x["types"], sep = ";")
return(t)
}
output = apply(vhl_variants_final, 1, format_string)
# Write the output to a file
write(output, file="CIViC-VHL-Variants.formatted.csv")
- Open the resulting file (CIViC-VHL-Variants.formatted.csv) in a text editor.
- Then, in ProteinPaint load the VHL gene.
- Use the + button to add data (top center), and choose the
SNV/Indel
option. - Then paste the formatted variant lines from the R cleanup exercise into that box and hit
submit
.
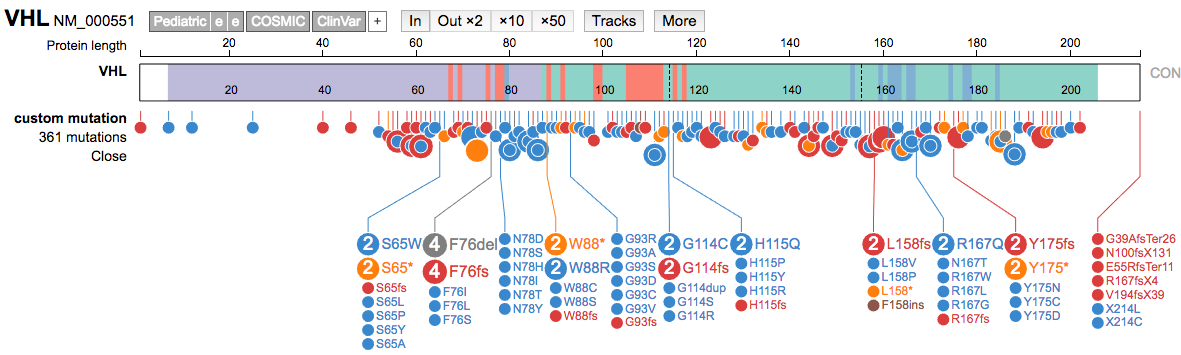
- Compare the VHL variants from CIViC to those in ClinVar.
ProteinPaint practice exercises
What are the three most recurrent mutation in PIK3CA according to COSMIC?
Get a hint!
Load PIK3CA, activate the COSMIC track, and look for the mutations with highest patient counts
Answer
H1047R, E545K, and E542K are the most recurrent mutations in PIK3CA according to COSMIC
What is the top tissue of origin observed for each of these three mutations?
Get a hint!
Click on the circle for each mutation and examine the tissue distribution plot
Answer
H1047R (breast), E545K (large intestine), and E542K (large intestine)
Load the Pediatric data for RUNX1T1. (A) What special kind of variant is indicated? (B) Load the RNA-seq plot for these data. Mouse over the RUNX1 variant. What interesting pattern do you observe? (C) Highlight the top 25 samples in the RNA-seq expression plot. What type of cancer dominates?
Get a hint!
Load RUNX1T1, make sure the Pediatric data track is activated, and make sure the RNA-seq gene expression panel is open
Answer
(A) RNA gene fusion variants. (B) The RUNX1-RUNX1T1 (aka AML-ETO) fusion variant corresponds to samples with very high RUNX1T1 expression. (C) AML cancer dominates the top 25 samples with highest RUNX1T1 expression.
Repeat the exercise above where we extract variants from CIViC for KRAS, create a clean version of these data, and load them into ProteinPaint.
Get a hint!
You should be able to do almost exactly what we did with VHL, but for KRAS instead
Advanced exercise. Identify a set of variants from your own gene for a single gene. Repeat the exercise above using these variants. If they are not human variants, it may be possible to first identify the closest human ortholog, and second to do “lift over”” of the coordinates.
Get a hint!
Depending on the form of the variants, some different kind of parsing and reformatting may be needed. If you need to convert the variants from one species to another you will learn more about tools for identifying orthologs and performing liftovers in later sections of this workshop. You may want to come back to this exercise...